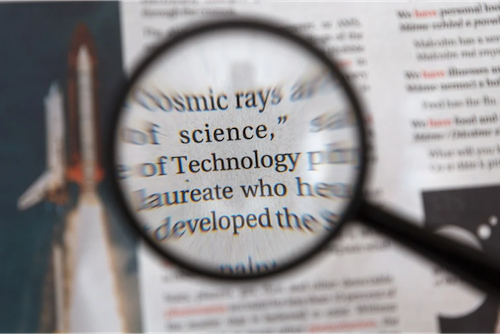
Behind the Words – From Spam Detection to Clinical Application of Text Analysis
Writing this blog post tells you, the reader, something about me, the author. Wouldn’t you agree? Obviously, it will give you information about my opinion on the topic of this blog post. But a written text incorporates more than isolated information on a certain topic. It can tell you something about me as a person. Am I confident? Am I educated? Am I enthusiastic about the topic I am writing about? Although I may lack conscious intentions to share such information to you, this sort of information is nonetheless entailed in form of word choice, text structure, and text dynamics.
Written words can provide us with personal information about the author beyond the actual written content.
So, what’s my point? In essence: Written words can provide us with personal information about the author beyond the actual written content. A comparable situation is when you look at a person for the first time. Beyond the obvious physical characteristics such as gender, height, or hair color you get information that can go beyond these objective traits. For example, you might notice things such as whether the person looks you in the eyes, smiles at you, has an upright body posture. All of these can tell you something about the person, assuming you pay attention to them. The same is true for written texts.
Just to clarify, I am not suggesting that by simply reading a randomly written text of a random person we are able to know everything about the author’s personality. That’s of course unrealistic. Rather, I suggest that the selection of specific words (e.g., “sad”), combination of words (e.g., “I am never sad”), and word groups (e.g., absolutist words such as “completely”, “definitely”, or “always”) can be indicative of psychological constructs that go beyond the word/s itself. For example, research on text analysis discovered that compared to non-depressed individuals, depressed individuals use fewer positive words, more first-person singular pronouns (i.e., self-referent words; e.g., Brockmeyer et al., 2015; Zimmermann et al., 2017) and more absolutist words (Al-Mosawi & Johnstone, 2018). In other words, the typical negative view of depressed individuals on life seems to manifests itself in form of a more or generalizable “depressive writing style”.
This may be academically interesting, but it is pointless without a practical application. The question remains how this can have practical value in the clinical setting? Well, it’s 2021 and you may have heard of the possible solution. It’s called machine learning algorithms.
A machine learning algorithm is a method by which an AI system predicts output values from a set of input data (e.g., written texts). The primary aim of these algorithms is classification. That is, based on the group characteristics derived from previously collected data, the algorithm automatically classifies new data from a new person into the categories of interest (e.g., Rosenbusch, Soldner, Evans, & Zeelenberg, 2021).
A machine learning algorithm is a method by which an AI system predicts output values from a set of input data (e.g., written texts).
Let’s make this easier with a familiar example: E-mail spam detection. Every e-mail you receive runs through a machine learning algorithm that decides whether an e-mail is more likely to be an important e-mail of, let’s say your supervisor, or a spam mail of, for example, from a gambling homepage. For a text classification algorithm to work we first need to train it. Sticking with the spam example, this means we need to gather as many e-mail texts as possible and feed them into the algorithm. Many algorithms that classify texts work on the basis of features, which, in its simplest form, are frequencies of single words (e.g., “money”), word combinations (e.g., “You won money.”), or specific word groups (e.g., first-person singular pronouns such as “I”, “me”, or “myself”) in proportion to the text lengths. During the training phase, the algorithm tries to identify features that best differentiate between spam and non-spam e-mails. Obviously, the more data that are available the more features that can be identified. After the algorithm has been trained and achieves a good classification accuracy (the percentage of classifying the text into the correct category) of the available e-mails, we can now use it to classify new e-mails for which we don’t know whether it is important or just spam. In fact, that’s all that such an algorithm does: Compare the characteristics of a new e-mail to the characteristics of many spam versus actually relevant e-mails.
Based on the same mechanism, a machine algorithm for depression detection could work. We “simply” need to have a lot of texts from the context of interest and identify the most relevant features to classify future texts. For example, in recent years more and more patients use different formats of online therapy, where patients do different exercises in a written format or correspond with their therapist via e-mail. These “data” might then be automatically analyzed by an algorithm to monitor changes in writing style. This in turn, could then help the online-therapist to identify individuals who do not benefit from treatment or might even worsen over the course of treatment. Hence, such a machine learning algorithm could act as an additional warning system, which does not require extra time on the side of the therapist. Despite the possible benefits in clinical psychology, it should not be neglected that such an algorithm can be a powerful tool when used in unintended ways. For example, do we want that insurance companies are potentially able to scan our social media post to determine whether we are at risk for mental disorder? Probably not!
…we should think carefully about how these might be applied in unwanted contexts and how we want to regulate this as a democratic society.
To sum it up, written texts can yield interesting information about the author’s personality and current emotion state. This information can potentially be extracted by machine learning algorithms and utilized for different purposes, for good (i.e., treatment) and for bad (e.g., insurance companies). Therefore, we should think carefully about how these might be applied in unwanted contexts and how we want to regulate this as a democratic society.
References
Al-Mosaiwi, M., & Johnstone, T. (2018). In an absolute state: elevated use of absolutist words is a marker specific to anxiety, depression, and suicidal ideation. Clinical Psychological Science, 7(3), 636–637. https://doi.org/10.1177/2167702617747074
Brockmeyer, T., Zimmermann, J., Kulessa, D., Hautzinger, M., Bents, H., Friederich, H.C., Herzog, W., & Backenstrass, M. (2015). Me, myself, and I: Self-referent word use as an indicator of self-focused attention in relation to depression and anxiety. Frontiers in Psychology, 6. https://doi-org.proxy-ub.rug.nl/10.3389/fpsyg.2015.01564
Rosenbusch, H., Soldner, F., Evans, A. M., & Zeelenberg, M. (2021). Supervised machine learning methods in psychology: A practical introdRosenbusch, H., Soldner, F., Evans, A. M., & Zeelenberg, M. (2021). Supervised machine learning methods in psychology: A practical introduction with annotated R code. Social and Personality Psychology Compass, 15(2). https://doi-org.proxy-ub.rug.nl/10.1111/spc3.12579uction with annotated R code. Social and Personality Psychology Compass, 15(2). https://doi-org.proxy-ub.rug.nl/10.1111/spc3.12579
Zimmermann, J., Brockmeyer, T., Hunn, M., Schauenburg, H., & Wolf, M. (2017). First‐person pronoun use in spoken language as a predictor of future depressive symptoms: Preliminary evidence from a clinical sample of depressed patients. Clinical Psychology & Psychotherapy, 24(2), 384–391. https://doi-org.proxy-ub.rug.nl/10.1002/cpp.2006
Picture credits
https://pixabay.com/de/photos/konzept-dokument-fokus-schreiben-18290/