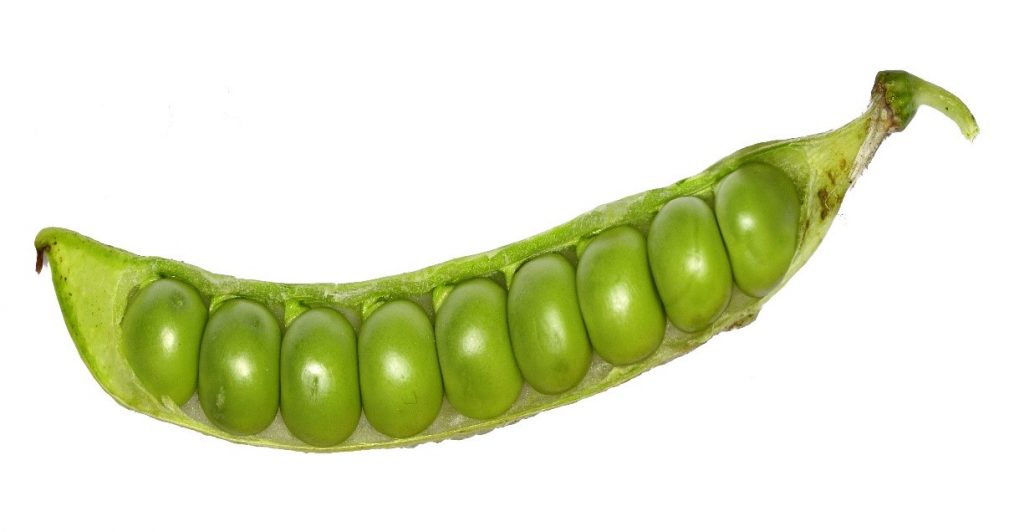
All we are saying is give p’s a chance
Methodologists had an interesting summer this past year, thanks in part to a bombshell paper by Benjamin and 71 others, shared as preprint on July 22nd, 2017. The authors argued to reduce the ‘default threshold’ α for statistical significance from 5% to 0.5% (i.e., from 0.05 to 0.005).
To refresh your memory, null hypothesis significance testing (NHST) works as follows:
- Postulate a null and alternative hypothesis (H0 and HA);
- Collect your data;
- Compute (using software) the p-value based on it;
- If p < α, reject H0; if p > α, don’t reject H0.
The p-value is the probability of finding a sample result as extreme or more extreme than the current result, given that H0 actually is true. This implies that when there is no effect (that is, when the H0 is true), there is a probability α of (incorrectly) rejecting H0. When the α level is set to 5%, which is custom the social sciences, this means that—when there was actually no effect—there’s a 5% chance that you will incorrectly claim to have found one: a false positive.
If you perform a study and find a significant result, it is difficult to find out whether this result is ‘real’ (a true positive) or ‘coincidence’ (a false positive). There are two-ways to gain additional information about which may be the case:
- Simply do the study again, based on another sample. If your original result was a false positive, it is very unlikely that your new result will again be significant. (And if you still unsure, just do the study again, and again, and again….) In the past few years, several large-scale studies—most notably OSF’s Reproducibility Project—have done exactly this, and found that roughly only one-third of significant results ‘replicate.’
- If you make educated guesses about (i) the probability that H0 actually is true, and (ii) the statistical power of your experiment, then you can estimate the probabilities of a false positive, a false negative, a true positive, and a true negative. You can then estimate the false positive rate (FPR), or the estimated proportion of significant results that are false positives.
Method 1 requires a lot of work: you need to re-do many studies. Benjamin et al. (2017) focused on method 2. This, however, has a drawback: you have to estimate numbers without really knowing if your estimates are any good.
Benjamin showed that, for certain reasonable conditions, the FPR will be as large as 50% if α = 5%, which is to say that half of the significant findings are actually false positives. When working with α = 0.5%, however, this FPR drops to 9%. Intuitively, this is also clear: if you make it much more difficult to claim a significant effect, it will be much less likely that you incorrectly claim a significant effect. This is the main reason why Benjamin et al. suggest lowering the default alpha-threshold by a factor of 10.
This sounds great. Let’s do it!
However, there is a trade-off between false positives and false negatives: by shifting the boundary between ‘significant’ and ‘non-significant’, we reduce the FPR but increase the false negative rate (FNR). In other words: more often than before, we would fail to label a true effect as significant. This is obviously a problem too.
You can compensate for the higher FNR by increasing statistical power. For this, you need to increase the sample size. But it has to go up by a lot: you’d need 70% to 88% more participants for your study. That new standard would then eliminate a lot of labs from less-wealthy universities. And this of course comes with further problems (like the association between wealth and WEIRDness).
It’s no surprise that Benjamin’s paper received criticism from various sources. Some authors (Amrhein, Greenland, 2017; McShane et al., 2017) suggested to stop after the third step: if you don’t draw conclusions, then you never draw false conclusions. According to them, it’s not the scientist’s task to decide whether the evidence to reject H0 is strong enough. We, however, thought that this advice is impractical: sometimes you just have to make a decision.
This ‘we’ is a team of 84 people, led by Daniël Lakens. In a nice example of open and transparent science, we’ve been working on a reply in a publicly-accessible Google Document. At the time of writing, this working document contains 110(!) pages of carefully considered arguments, which was then reduced in our reply to Benjamin et al. (2017) to about 18 pages.
In this reply, we outline why holding onto a default α-level—whether it is 5%, 0.5%, or something else that we might also agree-upon and then accept as a new convention—is not a good idea. Instead, we argue that the choice should always be carefully considered. Indeed, the chosen α-level should be informed by the context.
Consider the following two situations:
- You’re studying for an exam and you want to study efficiently. You want to put in sufficient hours to pass the exam, but also no more than that: you’re happy with a 6 and you would rather put the rest of your time in studying for other courses. If you fail the exam, you can do the resit in two weeks.
- Alas, you failed the exam. You’re now studying for the resit and still want to study efficiently (you have other things to do too.) But if you fail the exam this time, then you have to wait until next year for another attempt. And this study delay costs you another year of tuition fees, as well as the ire of your parents.
In both cases, a false positive would be thinking you had spent enough effort in the course, while still failing the exam. It is clear that the consequences of that are much bigger in the second example than in the first, so you will decide in advance to put in some extra effort to make sure that the false positive probability is smaller in case 2 than in case 1.
You should set your α to a stricter level when the stakes are higher.
The same line of reasoning holds in NHST. You can interpret your α-level roughly as “How bad is it if I accidentally (and incorrectly) call for an effect in this study?” It is not logical to give the same answer (α = 5%) to this question in every situation. You should justify your decision.
When the stakes are high, use a small α. When they are low, use a larger one.
This doesn’t sound like a groundbreaking suggestion. But that’s because it isn’t: mathematical statisticians have been saying this since the birth of NHST.
More than 50 years ago, Sir R. A. Fisher himself said much the same thing: “no scientific worker has a fixed level of significance at which, from year to year, and in all circumstances, he rejects hypotheses; he rather gives his mind to each particular case in the light of his evidence and his ideas” (see Lakens et al., 2017, p.14). As the urge is so strong to hold on to default values, rather than to put in an effort to motivate specific choices, it is good to remember where the defaults came from.
Our p’s are contemporary conventions, and nothing more. We don’t need to ban them. We just need to be a bit smarter about how we use them.
References
Amrhein, V., Greenland, S. (2017). Remove, rather than redefine, statistical significance. Nature Human Behaviour, paywalled at doi: 10.1038/s41562-017-0224-0
Benjamin, D.J., Berger, J., Johannesson, M., Nosek, B. A., Wagenmakers, E-J., Berk, R., …, Johnson, V. (2017, July 22). Redefine statistical significance. Preprint: doi: 10.17605/OSF.IO/MKy9J. Postprint in Nature Human Behaviour.
Henrich, J., Heine, S. J., & Norenzayan, A. (2010). Most people are not WEIRD. Nature, 466, 29. Shared via author home page at doi: 10.1038/466029a
Lakens, D., Adolfi, F. G., Albers, C. J., Anvari, F., Apps, M. A. J., Argamon, S. E., … Zwaan, R. A. (2017, September 18). Justify Your Alpha: A Response to “Redefine Statistical Significance”. doi:10.17605/OSF.IO/9S3Y6
McShane, B. B., Gal, D., Gelman, A., Robert, C., Tackett, J. L. (2017). Abandon statistical significance. Preprint at https://arxiv.org/abs/1709.07588