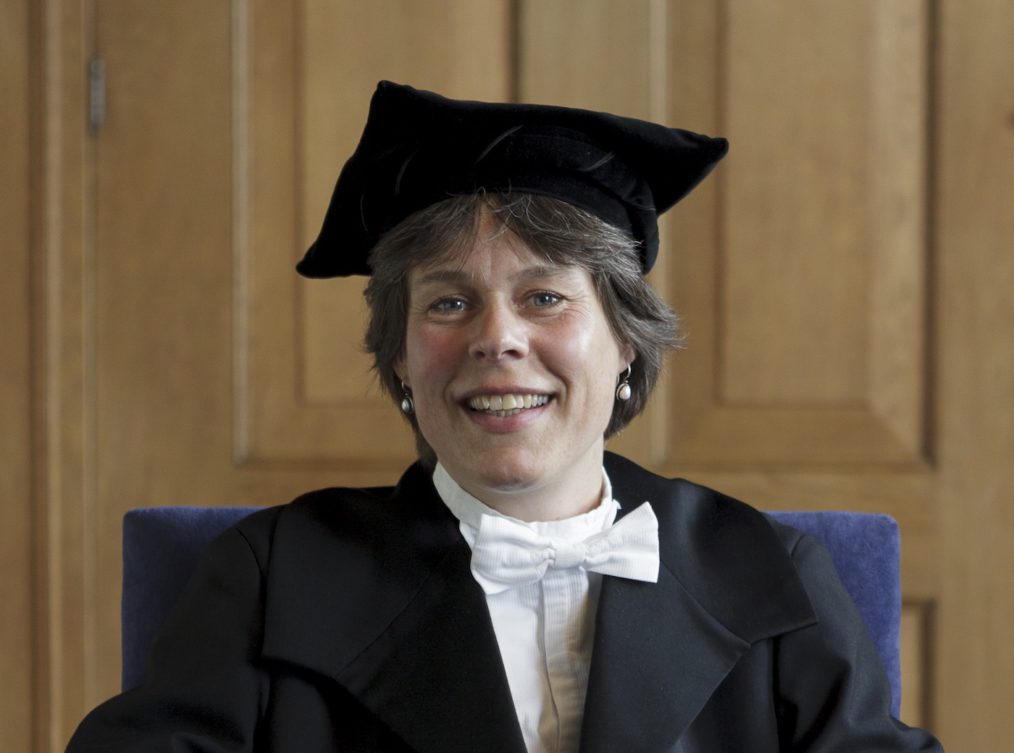
Gaining understanding with exploratory statistical modeling
Last month, Marieke Timmerman gave her Inaugural Lecture at the University’s Academy building, laying out her academic vision and presenting an overview of her research work. We asked her to write an article inspired by her lecture, so we can share it with Mindwise readers. – ed.
Psychology seems to be in crisis. Alarming messages are spread about the lack of reproducibility of findings reported in the literature. The media spread around the sobering results of the ambitious Reproducibility Project, in which researchers tried to replicate the findings from 100 psychology studies that were published in prominent psychology journals. Only a meagre 39 studies could actually be replicated (Open Science Collaboration, 2015).
The findings are shocking, as they give rise to doubts on the foundations of psychological theories. They yielded, and still do, intense debates in the Psychology community. Various factors contributing to these problems have been identified, ranging from the incentive system to a lack of proper use of methodology and statistics. To remedy the latter, methodologists argued strongly in favour of defining strict hypotheses before data collection, deciding about the statistical tests to apply in due course, and what to conclude on the basis of the associated results (Wagenmakers, Wetzels, Borsboom, van der Maas, & Kievit, 2012). This strategy perfectly applies to purely confirmatory research, but it falls short as soon as the research becomes more exploratory in nature.
Deliberately, I choose the term more exploratory, rather than adhering to the common distinction between strictly confirmatory and exploratory types of research (De Groot, 2014). All empirical research in psychology builds upon earlier observations, notions, ideas, theories, and empirical results. This implies that background knowledge is used in defining the objectives of the study and building the expectations about the results. The latter may be strong, resulting in strict hypotheses, or weaker, and very often one finds combinations of stronger and weaker expectations in the same study. In my view, these combinations are vital for deepening our understanding and expanding our knowledge. Confirmatory research seems to keep you on the safe side a low risk of false findings , but also prevents you from gaining exciting visions.
Now, the key issue is what a proper analysis strategy is in the absence of strict hypotheses. For sure, one needs to stay away from hypothesising after the results are known (so-called HARKing by Kerr, 1998), which boils down to just trying out various statistical analyses to trace “the most interesting and promising aspects of the data” (Sijtsma, 2015). Such an approach is dangerous, as one runs a serious risk of coming up with incorrect and non-replicable results.
But what approach is to be advised for more exploratory research? It is of key importance to write a solid research plan, including the objectives, research questions, design and analysis. In this respect the strategy is similar to what is required in a confirmatory study. What differs is that the proposed analysis will be more of an exploratory nature. Often, the analysis will include various steps to arrive at an interpretable statistical model of the data. Herewith, it is essential that the proposed analysis matches the objectives, the type of data to be collected and all available knowledge which directly indicates why it is so important to have a good overview of the available knowledge on the topic at hand. And, of course, to achieve a proper match one needs a thorough understanding of the statistical model itself.
An example illustrates the issue. It is known that prematurity at birth is a risk factor for lower levels of functioning in childhood. Of course, one could perform a confirmatory study, administering test X, which is indicative for a particular aspect of functioning, among random samples of children born preterm and at term, all at the age of Y. Then, the null-hypothesis would be that “average scores on test X are equal among the children born preterm and at term at the age of Y”, which can be tested with some sort of suitable t-test. There is nothing really wrong with testing this hypothesis, but it fails to provide really interesting insights, such as whether all preterm children would be affected similarly, whether all aspects would be equally affected, or whether there are protective factors. Using an exploratory statistical modeling, it was found that actually a large part of the children born moderately preterm showed functioning within the normal range, and only a minority below the normal range, while particularly boys appear at risk (Cserjesi et al., 2012). More exploratory approaches are very often really worth the effort, as one can achieve insights that remain kept hidden otherwise.
References
Cserjesi, R., Van Braeckel, K. N. J. A., Timmerman, M. E., Butcher, P. R., Kerstjens, J. M., Reijneveld, S. A., Bouma, A., Bos, A. F., & Geuze, R. H. (2012). Patterns of functioning and predictive factors in children born moderately preterm or at term. Developmental Medicine & Child Neurology, 54(8), 710-715. doi:10.1111/j.1469-8749.2012.04328.x
De Groot, A. (2014). The meaning of “significance” for different types of research [translated and annotated by E. Wagenmakers, D. Borsboom, J. Verhagen, R. Kievit, M. Bakker, A. Cramer, D. Matzke, G.J. Mellenbergh, and H.L.J. van der Maas]. Acta Psychologica, 148, 188-194.
Kerr, N. L. (1998). HARKing: hypothesizing after the results are known. Personality and Social Psychology Review : An Official Journal of the Society for Personality and Social Psychology, Inc, 2(3), 196-217. doi:10.1207/s15327957pspr0203_4 [doi]
Open Science Collaboration. (2015). PSYCHOLOGY. Estimating the reproducibility of psychological science. Science (New York, N.Y.), 349(6251), aac4716. doi:10.1126/science.aac4716 [doi]
Sijtsma, K. (2015). Playing with data—Or how to discourage questionable research practices and stimulate researchers to do things right. Psychometrika, , 1-15.
Wagenmakers, E. J., Wetzels, R., Borsboom, D., van der Maas, H. L., & Kievit, R. A. (2012). An Agenda for Purely Confirmatory Research. Perspectives on Psychological Science : A Journal of the Association for Psychological Science, 7(6), 632-638. doi:10.1177/1745691612463078 [doi]