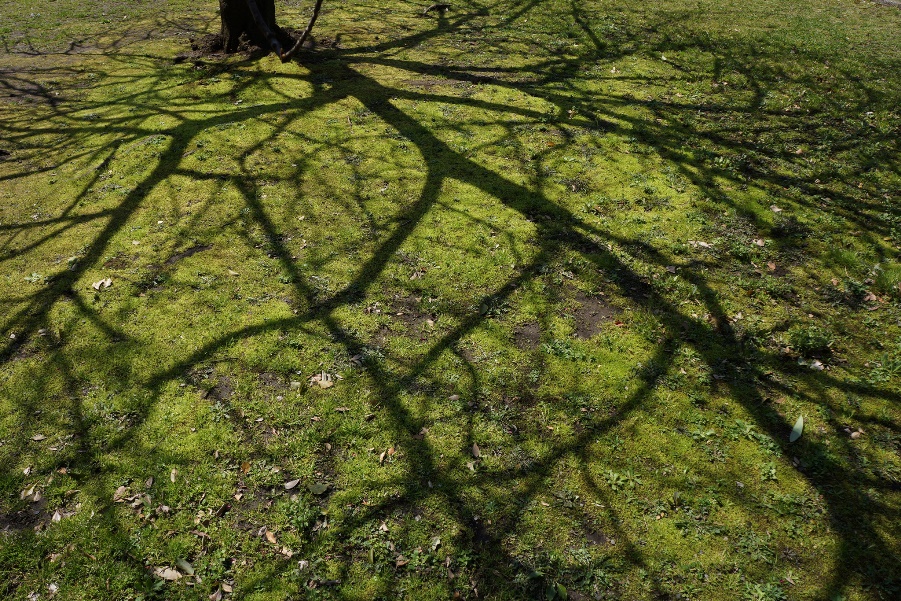
Reflections of an open science convert 1: Why I changed my research practices
(This is part 1 of a 3-part series. Part 2 can be found here and Part 3 here)
As a Master-level student I worked with a young and ambitious assistant professor at the University of Toronto. Sadly, Professor Z. and I did not get along very well, probably because of my Dutch directness and insensitivity to hierarchy, I thought. Three years later, however, it turned out that she had manipulated multiple datasets, including the data I had collected when I worked with her. I was appalled. Suddenly it made a lot of sense why working with her had been so difficult. However, this happened in the 1990s and for me the consequences were far from earth-shattering (though they were for Professor Z., who was fired). I adopted the joke that “z-transformation” had gained a different meaning and carried on with my PhD project.
About 15 years later I was on the promotion committee of one of Diederik Stapel’s PhD students. I asked a question about a result that later turned out to be based on fabricated data. When I found out about the data fabrication, again I was appalled: I had not been able to tell fake from real at all. I had not even considered the possibility that the data might be fake. However, the magnitude, deliberateness, and shamelessness of Stapel’s fraud seemed such an exception that I could think of nothing to take home from it. So I carried on with my research in experimental psychopathology/clinical psychology, hardly paying attention to what was going on in adjacent areas of psychology.
“I had not been able to tell fake from real at all.”
Sure, I heard about p-hacking. I just did not think that it applied to me. After all, I was cautious, anxious even, to write up my studies as accurately as possible. Among all the data analytic possibilities that presented themselves, I was particularly critical of the ones that easily yielded p < .05. Often the results were difficult to interpret and I did not trust my data-analytic approach (Surely there must be a better way of remediating this awkward residual plot?). Indeed, a different approach would then lead away from statistical significance. This, together with doubts about the research method (Everybody else finds this effect, surely I must be doing something wrong!), resulted in a stack of unfinished papers in my file drawer.
Five years after Stapel’s fraud first became known, I came across Brian Wansink’s blog posts about how exploring data in every possible way can get you publications. The scientific community responded with outrage. On the one hand, Wansink’s data-dredging seemed far more extreme than the post-hoc analyses I used to do. On the other hand, I wondered what exactly, apart from the scale (huge) and intent (find a positive result no matter what), the differences were between me and him.
“precisely one of those rare statistically significant occurrences had made it into the literature”
So I wanted to find out what exactly is wrong with deciding which analytic route to take based on the data. As I browsed the internet, an online lecture by Zoltan Dienes caught my attention. Dienes described the problem that Gelman & Loken (2014) refer to as the garden of forking paths: the idea that every potential, often seemingly arbitrary decision in data analysis (e.g., how to construct a score; what to do with outliers) contributes to a different end-result. Indeed, it is like hiking: choosing either left or right at the first fork in the path (and the fork after that, and the one after that, etc.) will determine where you will have lunch ultimately. Dienes used the example of one particular published study that implicitly harboured 120 plausible combinations of decisions (1). A plot of the 120 possible difference scores for one particular variable (i.e., a multiverse) showed that their confidence intervals could contain exclusively positive as well as exclusively negative values, and mostly hovered around zero (i.e., no difference). Thus, despite what seemed a convincing effect in the paper, considering the full array of outcomes for that one variable should lead to the conclusion that really nothing can be said about it.
I was stunned. So many possibilities, and precisely one of those rare statistically significant occurrences had made it into the literature! Perhaps by coincidence, perhaps because certain routes fit better with the authors’ hypothesis than other routes? But regardless of why this particular result ended up in the paper, how can readers even know about those other 119? In addition to stunned, Dienes’ lecture left me horrified. I realized that even though I had been motivated to steer my decisions away from the paths that would yield significance easily, the very steering with a direction in mind would yield conclusions that were just as likely to be biased as in Dienes’ example.
“I am working on changing my research practices”
So, now I am working on changing my research practices. No multiverse analyses (yet), but I try to protect myself against post-hoc bias by preregistering new studies before collecting data. There are different formats for doing so, and I like the one that requires me to be as detailed as I can. I find that hugely beneficial. Not only do I need to spell out the method (When to stop collecting data? How to construct a score given the various possibilities for this particular measure?), but also I need to figure out which analysis I will use given various alternatives. Determining what to do in advance and staying with it saves me a lot of time and doubt later. There is an end-point. That doesn’t mean I don’t do exploratory analyses anymore. But preregistering helps me to be clear about what I promised myself I would do and what might be interesting other avenues to venture into.
Another (related) way in which I try to find my way in the garden of forking paths is by increasing transparency and openness. I am aware that this does not solve the problem that my conclusions are based on only one set of decisions among multiple, equally plausible sets of decisions. However, being transparent about the route that led to a particular result should help others evaluate the value of the outcome. In addition, I hope that sharing my data openly enables others to understand the underpinnings of my claim better, and perhaps explore questions of their own with them. It can even help avoid publishing mistakes. So now I aim at posting all pre-registrations, study materials, and data in publicly accessible repositories, insofar as copyright and privacy laws permit. In addition, because I have come to believe that sharing should be common practice, I signed the Peer Reviewer Openness (PRO) initiative. PRO-signatories strive for more transparency by making comprehensive manuscript reviews conditional on open data and materials.
“I have come to believe that sharing should be common practice”
So, all’s well that ends well, and I live happily ever after? Not exactly. In fact, I have found it hard to maintain my new research practices in a community that mainly relies on “old-school” reflexes. In parts 2 and 3 of this blog series, I will tell you why.
(1) Dienes used the example discussed in Steegen, Tuerlinckx, Gelman & Vanpaemel (2016).
References:
Gelman, A., & Loken, E. (2014). The Statistical Crisis in Science. American Scientist, 102(6), 460–465. doi:10.1511/2014.111.460
Steegen, S., Tuerlinckx, F., Gelman, A., & Vanpaemel, W. (2016). Increasing transparency through a multiverse analysis. Perspectives on Psychological Science, 11(5), 702–712. doi:10.1177/1745691616658637
Note:
Image by Zengame, licenced under CC BY 2.0
One comment