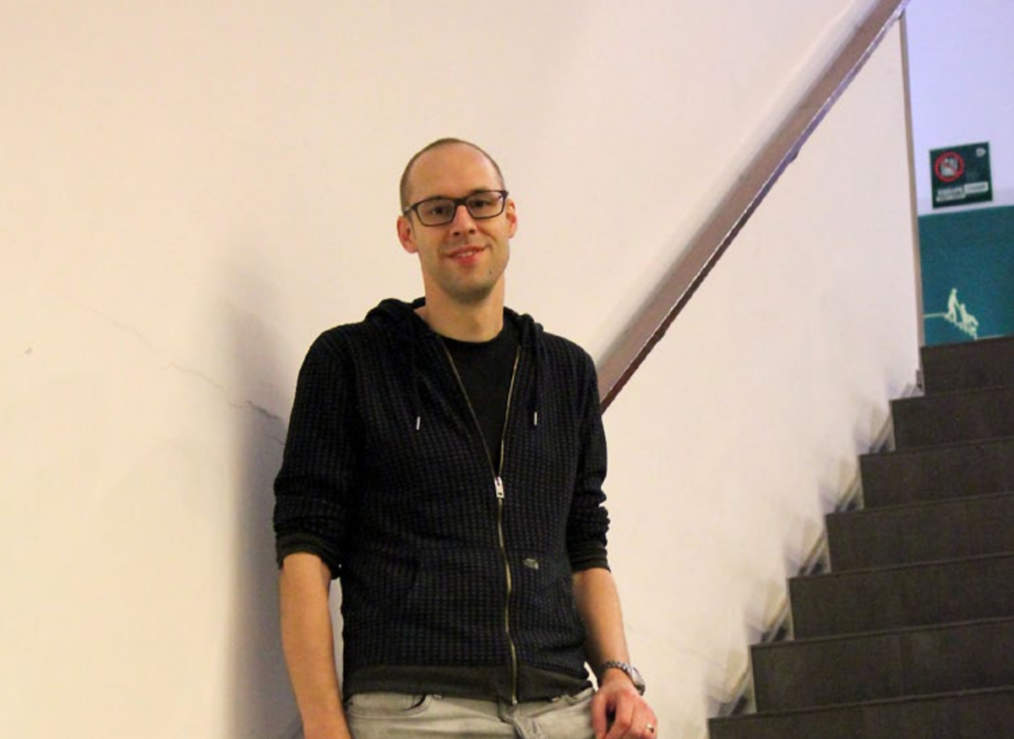
On Track to Read Your Mind?
An Interview with Jelmer Borst
Recently, Jelmer Borst received a prestigious NWO VENI grant to investigate how people process complex issues, like an algebra problem, in their brains. To gain more insight into this phenomenon, he aims to develop a detailed computational model of problem solving in the fronto-parietal network to uncover more about general problem-solving mechanisms. Michiel Hooiveld, Research Coordinator at BCN-Brain, interviewed him for the latest issue of the BCN Newsletter.
Michiel Hooiveld: What approach will you choose within your research project to uncover more about problem solving in the fronto-parietal network?
Jelmer Borst: I will use two innovative analysis techniques that I helped develop: model-based fMRI analysis and Hidden semi-Markov Model (HSMM) analysis. I will use both techniques to analyse data of the same experiment measured with three different neuroimaging methods (fMRI, EEG, and MEG).
MH: What is so unique about these two techniques in combination with these three different neuroimaging methods?
JB: Model-based fMRI analysis and HSMM analysis are more powerful than traditional neuroimaging analysis methods. In model-based analysis, we use a computer simulation of the task as the basis of our data analysis, which results in superior localization compared to standard methods, as well as providing a direct link between theory and data. However, this analysis is dependent on the quality of the models that we use. I will therefore also use a new method that combines multi-variate pattern analysis with hidden semi-Markov models to automatically discover cognitive processing stages – and thereby the basis for a computer model – in fMRI or MEG/EEG data. So, I will approach the problem from two sides: on the one hand I use model-based analysis to do theory-guided research, while applying the MVPA-HSMM method to also approach the problem in a bottom-up manner.
In addition, using three different neuroimaging methods for a single experiment provides a unique opportunity to combine the strengths of these methods: fMRI has high spatial resolution, while EEG and MEG have excellent temporal resolution, and MEG reasonable spatial resolution. The data of these three methods, in combination with the new analysis techniques, will hopefully allow me to build a detailed computational model that explains how problem solving works in the brain.
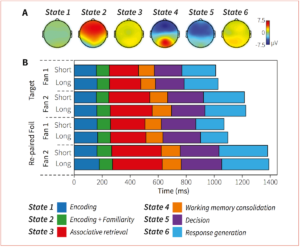
Results of the HSMM analysis. A) shows the brain signatures of six different processing states that were identified in EEG data of an associative recognition task. B) shows the duration of these six states in the different conditions. This indicates, for instance, that the ‘Associative retrieval’ state determines for a large part the variability of the response times, while occurring early in the trial. Adapted from Borst & Anderson, 2015, NeuroImage.
MH: What would you ultimately like to achieve within the framework of your project?
JB: On a theory level, I hope to construct a detailed computational model that explains how problem solving functions at an algorithmic level within the fronto-parietal loop. At the same time, model-based fMRI analysis and HSMM analysis are still very recent developments. I hope that my research contributes in developing these methods into mature analysis techniques. These days, it’s relatively easy to collect neuroimaging data, but I think we need better analysis techniques to get at all the information that is present in these data.
MH: What are the main applications of your results if your project succeeds?
JB: Besides providing insight into problem solving, I think the main applications will be the analysis methods. We already publish all the code and data together with our papers to enable other researchers to use our methods, and one thing I would like to do is move the code from Matlab to open source languages (e.g., Python, R) to make it more accessible.
MH: Are we really on track to read people’s minds in the near future?
JB: My research is aimed at improving model-based fMRI and HSMM analysis and developing them into mature analysis techniques. I am convinced that this will bring us one step closer to true mind reading.
Neuroimaging MethodsfMRI estimates neural activity by measuring blood oxygenation. The measured signal follows neural activity by 4-6 seconds. fMRI’s spatial resolution is in the order of millimeters.
EEG measures the electrical signal of the brain on the scalp. As the skull distorts the electrical signal, its spatial resolution is low. However, its temporal resolution is excellent.
MEG measures the magnetic signal of the brain on the scalp. Because the magnetic signal is hardly distorted by the skull and brain tissue it is possible – albeit complex – to reconstruct the origin of neural activitywith a precision of ~1cm. MEG’s temporal resolution is excellent.
|
Curriculum Vitae
Jelmer Borst (1983) completed a bachelor in Artificial Intelligence at the University of Groningen, and the BCN research master. He received his PhD in Artificial Intelligence in 2012 from the University of Groningen, by developing computational models of behavioral and fMRI data of multitasking. From 2012 to 2014 he worked as a postdoctoral researcher at Carnegie Mellon University investigating new analysis techniques for fMRI and EEG data. In 2014 he moved back to the Artificial Intelligence department, where he started his VENI research project in January of this year.
Further reading