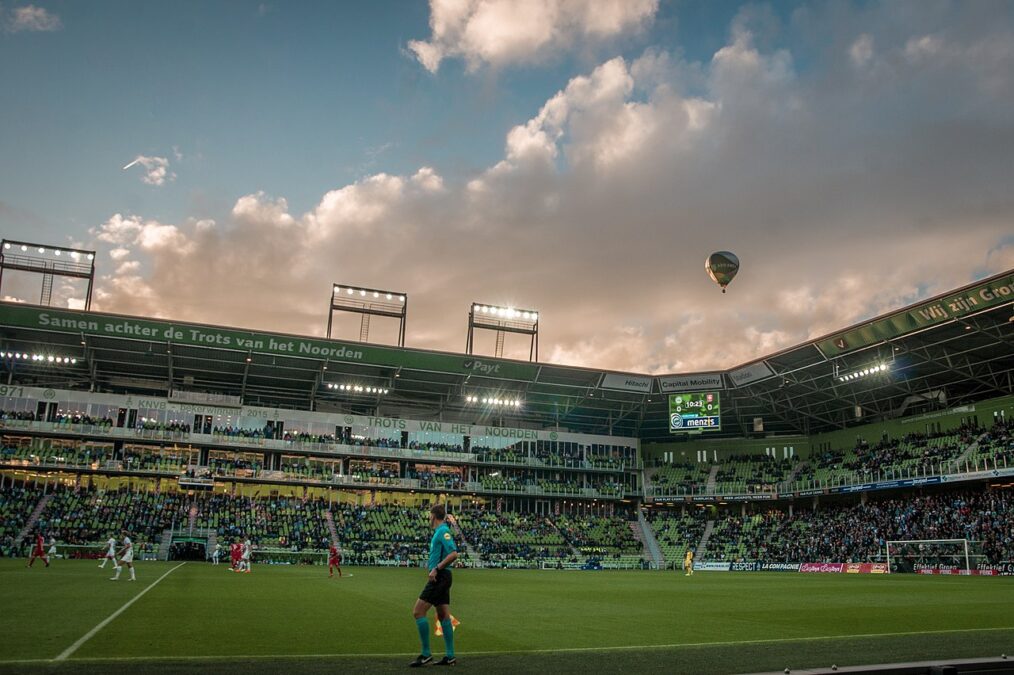
WHO IS AVERAGE ANYWAY? THE ROAD TO SUCCESS WITH A PERSONALIZED APPROACH
Imagine you are the former professional football player Arjen Robben. You have won several national and international trophies and are considered one of the best players of all time in Dutch football history. Today is training day. You wake up and get ready to go to the field. For a few days you have been feeling miserable because you have not slept well and your muscles are extremely sore. Like every morning before training, you answer questions about your recovery status on a tablet. Based on the results of all players, the coach usually adjusts the load (physiological stress, i.e., how hard the training is) of the session. Today, however, the rest of the team is doing great. Everyone else is well-rested and feels ready to perform. And since the team’s recovery average is quite high, the coach makes the choice to even increase the load of the session. You decide not to complain and stick to the schedule. You start the first exercise and after 30 minutes the worst-case scenario occurs: You get injured.
A clear takeaway from this fictional story is that training recommendations based on groups of athletes often have no logical bearing for the individual. If coaches design training sessions to fit the average athlete, ignoring meaningful individual deviation, they have actually designed them to fit no one. Or as Todd Rose would put it: “Any system designed around the average person is doomed to fail” (Rose, 2016, p.8).
Don’t get me wrong, group-level approaches are neither false nor bad. It simply depends on what we want to know and on which level (group or individual) we want to apply our insights from the data. This, in turn, paves the way for how data in research and practice is collected and analyzed. For instance, if we want to know whether athletes with high levels of load also tend to have high levels of performance, then the group-level analysis is best suited because we would sample across all individuals. This approach will increase our understanding of variables and how they are distributed across athletes (Bland & Altman, 1994; Van Geert, 2014).
“If we want to know whether athletes with high levels of load also tend to have high levels of performance, then the group-level analysis is best suited because we would sample across all individuals.”
However, in monitoring athletes, both theorists and practitioners are usually interested in how variables, such as the load, develop across time within single individuals. To be more specific, scientists want to understand these processes to provide evidence-based recommendations to practitioners. The practitioners wish to understand how they should coach their athletes to enhance sports performance and to decrease the likelihood of injuries (Neumann et al., 2021). This said, although researchers and practitioners are interested in applying their insights from the data to the individual athlete, data collection and analysis are yet predominantly conducted on a group level.
This is problematic, because recent research showed that findings on the group level are not generalizable to the individual (Hill et al., 2020; Neumann et al., 2021). For instance, we found that individual athletes displayed more variability than groups in measures such as load and recovery (Neumann et al., 2021). In the same line, correlations between those two variables were sometimes weaker or stronger in individuals than in groups.
“Recent research showed that findings on the group level are not generalizable to the individual (Hill et al., 2020; Neumann et al., 2021).”
Interestingly, this effect has been observed in other fields as well, such as medicine and the behavioral and social sciences (Blaauw, 2018; Fisher et al., 2018; Molenaar & Campbell, 2009). Bakdash & Marusich (2017), for example, showed that the common individual correlation between accuracy and response time in visual search is -.41, whereas it is .38 when all the data is put together as in a group approach.
A PARADIGM SHIFT: THE SCIENCE OF THE INDIVIDUAL
In contrast to the group-level approach, the individual-level approach seeks to reveal, for instance, whether changes in load are paralleled by changes in performance within an athlete. Hence, one needs to monitor individuals repeatedly and sample across consecutive measurement points. This approach will increase our understanding of processes within individuals.
By analyzing the repeated measures of individuals, one should aim at extracting meaningful information out of the data, including possible changes over time, instead of relying on averages (Bringmann et al., 2018; Mangalam & Kelty-Stephen, 2021). For instance, while the influence of load on performance may be positive in one month, it might turn negative in the upcoming month. An average relation between the variables would omit the possibility that the strength or direction of the effect has changed over time. This change could be interpreted as a warning signal for the coach to decrease the load for that specific individual. Ignoring the possibility of changing effects over time, or simply relying on (group) averages may have serious consequences for individual athletes, as they may be completely mistreated.
“By analyzing the repeated measures of individuals, one should aim at extracting meaningful information out of the data, including possible changes over time, instead of relying on averages (Bringmann et al., 2018; Mangalam & Kelty-Stephen, 2021).”
To conclude, one cannot understand individuals by ignoring their individuality (Rose, 2016, p.62). Researchers and practitioners are more likely to develop high-performing and healthy athletes when monitoring their individual-specific behavior over time. In that way, coaches may better determine when to intervene and which specific knob to turn for which individual. The road to success may thus lie in a personalized approach.
Links
- Ted Talk by Todd Rose about the myth of average: https://www.youtube.com/watch?v=4eBmyttcfU4
- Our project about a personalized approach to resilience monitoring and analysis in sports: https://project-ris.
nl/“
References
Bakdash, J. Z., & Marusich, L. R. (2017). Repeated measures correlation. Frontiers in Psychology, 8(456), 1–13. https://doi.org/10.3389/fpsyg.2017.00456
Blaauw, F. J. (2018). The non-existent average individual: Automated personalization in psychopathology research by leveraging the capabilities of data science. University of Groningen.
Bland, J. M., & Altman, D. G. (1994). Statistics Notes: Correlation, regression, and repeated data. British Medical Journal, 308(6933), 896. https://doi.org/10.1136/bmj.308.6933.896
Bringmann, L. F., Ferrer, E., Hamaker, E. L., Borsboom, D., & Tuerlinckx, F. (2018). Modeling Nonstationary Emotion Dynamics in Dyads using a Time-Varying Vector-Autoregressive Model. Multivariate Behavioral Research, 53(3), 293–314. https://doi.org/10.1080/00273171.2018.1439722
Fisher, A. J., Medaglia, J. D., & Jeronimus, B. F. (2018). Lack of group-to-individual generalizability is a threat to human subjects research. Proceedings of the National Academy of Sciences of the United States of America, 115(27), E6106–E6115. https://doi.org/10.1073/pnas.1711978115
Hill, Y., Meijer, R. R., Van Yperen, N. W., Michelakis, G., Barisch, S., & Den Hartigh, R. J. R. (2020). Nonergodicity in protective factors of resilience in athletes. Sport, Exercise, and Performance Psychology. https://doi.org/http://dx.doi.org/10.1037/spy0000246
Mangalam, M., & Kelty-Stephen, D. G. (2021). Point estimates, Simpson’s paradox, and nonergodicity in biological sciences. Neuroscience and Biobehavioral Reviews, 125, 98–107. https://doi.org/10.1016/j.neubiorev.2021.02.017
Molenaar, P. C. M., & Campbell, C. G. (2009). The new person-specific paradigm in psychology. Current Directions in Psychological Science, 18(2), 112–117. https://doi.org/10.1111/j.1467-8721.2009.01619.x
Neumann, N. D., Van Yperen, N. W., Brauers, J. J., Frencken, W., Brink, M. S., Lemmink, K. A. M. P., Meerhoff, L. A., & Den Hartigh, R. J. R. (2021). Nonergodicity in Load and Recovery: Group Results Do Not Generalize to Individuals. International Journal of Sports Physiology and Performance. Advance Online Publication. https://doi.org/10.1123/ijspp.2021-0126
Rose, T. (2016). The end of average: How to succeed in a world that values sameness. Penguin UK.
Van Geert, P. (2014). Group versus individual data in a dynamic systems approach to development. Enfance, 3(3), 283–312.
Note: Featured image Victor van Werkhooven, CC 3.0, via Wikimedia Commons